Introduction
As technological advancements continue to reshape industries, Artificial Intelligence (AI) is a pivotal force driving innovation and transformation. By 2025, AI will not only redefine job roles and processes but also open doors to new opportunities across sectors. To succeed in this dynamic environment, prioritizing upskilling with AI for career is no longer an option—it’s a necessity.
This guide delves into the critical skills, tools, and strategies required to harness AI effectively and secure your place in the workforce of the future.
1. The Growing Need for Upskilling with AI for career
1.1 The Impact of AI on Modern Careers
Artificial Intelligence is reshaping how industries function, driving efficiency, innovation, and decision-making. Fields such as healthcare, education, retail, and finance are integrating AI to streamline processes and generate new opportunities. For professionals, acquiring AI-related skills is no longer optional—it’s a pathway to staying relevant in an increasingly tech-driven world.
Advantages of AI Proficiency in the Workplace:
- Time Savings: Automated systems handle repetitive tasks, allowing for greater focus on critical projects.
- Strategic Thinking: AI tools provide insights that support informed and impactful decision-making.
- Career Expansion: Emerging roles, including AI integration specialists and automation consultants, are creating new possibilities.
- Borderless Career Paths: AI knowledge equips professionals to compete in a global job market where tech skills are in high demand.
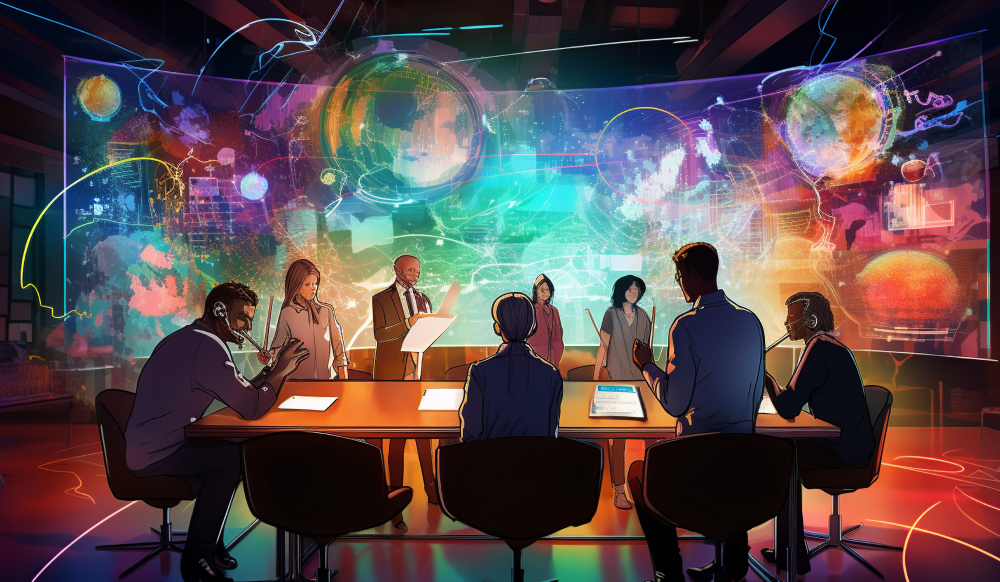
1.2 AI in the Job Market
AI is creating new job roles like AI ethicists, machine learning engineers, and automation specialists while enhancing traditional roles with data-driven insights. Upskilling in AI for career growth ensures relevance and competitiveness in this dynamic market.
Industries Impacted by AI:
- Healthcare: Revolutionizing patient care and diagnostics.
- Finance: Streamlining risk management and fraud detection.
- Retail: Enhancing customer experience through personalization.
- Manufacturing: Optimizing production with predictive maintenance.
2. Core Skills for AI for Career Growth
2.1 Technical Skills
To excel in AI, building a foundation in technical competencies is crucial:
- Programming Languages: Learn Python, R, or JavaScript for AI model development.
- Machine Learning (ML): Understand supervised and unsupervised learning, neural networks, and reinforcement learning.
- Data Analytics: Develop expertise in data cleaning, visualization, and interpretation using tools like Tableau or Power BI.
- Cloud Computing: Familiarize yourself with platforms like AWS, Google Cloud, and Azure for deploying AI solutions.
- Natural Language Processing (NLP): Gain knowledge in processing and analyzing human language data.
- Computer Vision: Understand AI techniques for image and video analysis.
2.2 Non-Technical Skills
Non-technical skills are equally vital for integrating AI into practical applications:
- Critical Thinking: Analyze complex problems and evaluate AI-driven solutions.
- Ethical Awareness: Understand the ethical implications of AI in decision-making.
- Communication Skills: Translate technical insights into actionable strategies for non-technical stakeholders.
- Project Management: Organize and oversee AI projects effectively, ensuring timely delivery.
3. How to Get Started with AI Upskilling
3.1 Assessing Your Current Skill Level
Begin by identifying gaps in your skillset:
Self-Evaluation: Map your existing skills to industry demands.
Feedback: Seek input from mentors or peers to understand areas of improvement.
Tools for Skill Assessment:
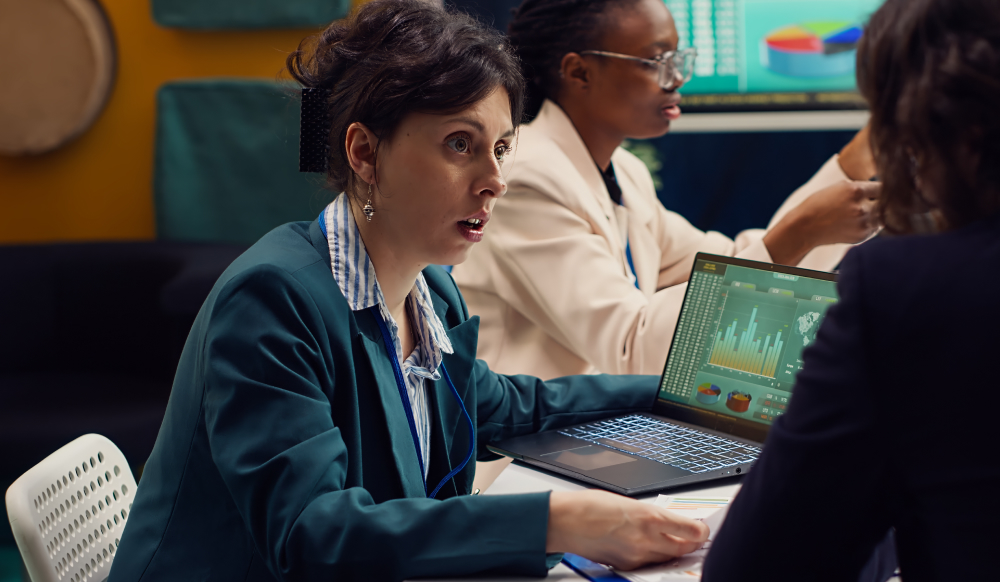
LinkedIn Skills Assessment Tests: Measure your expertise in AI and related fields.
Platforms like Skillshare or Pluralsight: Provide skill gap analysis tools.
3.2 Defining Your AI Learning Objectives
To succeed in your journey of mastering AI for career enhancement, it’s essential to set clear and actionable goals. By breaking down your ambitions into short-term and long-term targets, you can maintain focus and motivation throughout the learning process.
Examples of Goal Setting:
- Short-Term Targets: Dedicate three months to learning a foundational programming language like Python.
- Long-Term Aspirations: Aim to gain proficiency in designing and deploying AI models within a year.
Effective Goal-Setting Strategies:
- SMART Objectives: Create goals that are Specific, Measurable, Achievable, Relevant, and Time-bound to ensure clarity and progress.
- Progress Tracking: Use project management tools such as Trello or Asana to monitor milestones and stay organized.
4. Choosing the Right Learning Resources
4.1 Online Courses
Enroll in platforms offering AI and ML courses:
- Coursera: Offers certifications in AI fundamentals from top universities.
- edX: Features programs like MIT’s Artificial Intelligence course.
- Udemy: Provides affordable, beginner-friendly AI tutorials.
- DataCamp: Focuses on data science and machine learning courses.
4.2 Essential Reads for Expanding Your AI Expertise
To gain deeper insights into the world of Artificial Intelligence, consider these carefully curated resources:
“AI Systems Demystified” by Michael Negnevitsky: This book offers a beginner-friendly overview of AI concepts, making it ideal for those new to the field.
![]() | Mastering Deep Learning Techniques by Ian Goodfellow and others A comprehensive resource that explains the mechanics and applications of deep learning in modern technology. | |
![]() | Superintelligence and Its Implications by Nick Bostrom A compelling examination of AI’s potential to surpass human intelligence and the challenges it may bring. |
“A Beginner’s Guide to Machine Learning” by Andriy Burkov: Perfect for readers seeking a concise and practical understanding of machine learning fundamentals.
4.3 Communities and Forums to learn more about AI for career Development
Join AI-focused communities to stay updated:
- Participate in platforms like Kaggle for data science competitions.
- Engage in discussions on Reddit’s AI and ML subreddits.
- Join LinkedIn Groups focused on AI and emerging technologies.
4.4 Certifications in AI for career development
Earning industry-recognized certifications can validate your skills:
- Google AI Certificate: Comprehensive courses on machine learning.
- Microsoft AI Fundamentals: For beginners in AI.
- AWS Certified Machine Learning: Focuses on deploying ML solutions.
5. Applying AI Skills in the Workplace
5.1 Exploring Practical AI Applications
Understanding where AI can add value is key to integrating it effectively in your professional environment. Focus on areas that benefit from improved efficiency, automation, or advanced analytics:
- Streamlining Routine Tasks: Leverage Robotic Process Automation (RPA) to handle repetitive or time-consuming processes, freeing up time for strategic work.
- Enhancing Customer Insights: Use predictive analytics to analyze data and forecast customer preferences or market trends, enabling proactive decision-making.
- Improving Workflow Efficiency: Adopt AI-powered tools for managing schedules, allocating resources, or optimizing operational processes.
5.2 Collaborating Across Teams
Upskilling in AI enhances cross-functional collaboration:
- Data Teams: Work with data scientists to implement machine learning models.
- Business Leaders: Translate AI insights effectively to influence strategic decisions.
Tools for Workplace Application:
- AI-Powered CRM: Tools like Salesforce Einstein.
- Project Management AI: Platforms like Monday.com AI assistant.
- HR and Recruitment AI: Leverage tools like HireVue for data-driven hiring.
6. Industry-Specific AI Applications
6.1 Healthcare
- Predicting Patient Outcomes: AI algorithms forecast recovery probabilities.
- Diagnostic Accuracy: Computer vision tools enhance radiology assessments.
- Telemedicine: AI chatbots and tools facilitate remote consultations.
6.2 Finance
- Fraud Detection: ML models flag suspicious transactions in real time.
- Algorithmic Trading: AI systems optimize stock trading strategies.
- Risk Assessment: Automate credit scoring and risk profiling.
6.3 Retail
- Customer Personalization: AI recommendation engines enhance shopping experiences.
- Inventory Management: Predictive analytics prevent stockouts and overstocking.
- Dynamic Pricing: Adjust pricing strategies based on demand forecasts.
6.4 Manufacturing
- Predictive Maintenance: AI predicts equipment failures before they occur.
- Quality Control: Vision systems detect defects in production lines.
- Supply Chain Optimization: AI streamlines logistics and delivery processes.
7. Navigating Challenges to Upskill in AI for career growth
7.1 Overcoming Learning Barriers
- Modular Learning: Break down complex topics into manageable modules.
- Practical Application: Engage in hands-on projects to reinforce theoretical knowledge.
- Community Support: Seek mentorship and collaborate in AI-focused groups.
7.2 Addressing Ethical Concerns
- Understanding AI Ethics: Study case studies to grasp societal impacts.
- AI Governance: Learn about global regulations and compliance frameworks.
- Bias Mitigation: Develop skills to identify and reduce algorithmic biases.
8. Future Trends in AI and Upskilling
8.1 AI Democratisation
As tools become more accessible, upskilling efforts will focus on enabling non-technical professionals to harness AI effectively.
8.2 Focus on Responsible AI for career
Upskilling will increasingly emphasize ethical AI design and bias mitigation.
8.3 Lifelong Learning
Continuous learning AI for career will be essential as it evolves. Stay adaptable by enrolling in periodic training programs.
Emerging Tools for Lifelong Learning:
- AI Mentorship Platforms: Personalized learning pathways guided by AI for career progress.
- Real-Time Feedback Tools: Platforms that provide instant assessments on projects.
Also read: Bridging the critical AI Talent Gap: 6 Approaches to Hiring and Retaining Skilled Professionals
Leave a Reply